In Part-I we discussed advantages, security, and compliance consideration, challenges, and governance aspects of AI.
In part-II we focused on AI policies.
Here is part-III. We cover AI Use Cases and Data.
It’s not a surprise that every new cyber product we come across has something to do with machine learning (ML) and artificial intelligence (AI). The subject of AI is of significant interest and many organizations are likely to buy or have already bought AI products without understanding the larger implications of adopting AI. AI within the organization needs to be governed by policies and procedures, as well as other considerations, such as ethics, accountability, and transparency.
The use cases below are from the Government of the UK Office for Artificial Intelligence. This is about a signaling company using AI to help trains run on time. The company responsible for managing railway traffic wanted to predict when delays were likely to happen and use these insights to help controllers prepare for and minimize the impact on the train network. This would allow the company to redirect traffic in a way that reduced delays.
The company built the following three separate models which interact with one another.
- A forecasting model to predict delays using historical data which included features such as train arrival times, position, lateness, and the timetable
- A model to learn patterns in the data so controllers can better understand how the network operates and what causes delays
- A recommendation engine to suggest alternative platforms to controllers
The new system can give the signaling company warnings of delays up to an hour in advance with 50% higher accuracy than previously.
The other use case is using data from electricity meters to predict energy consumption. A research institution needed to understand which electric appliances were being used in a house at a certain time to optimize heating and energy consumption. The research institution used non-intrusive load monitoring to gather unlabeled data from electricity meters to see which appliances were being used and when.
They used unsupervised machine learning techniques to convert the unlabeled data into patterns. From this, the research institution could cluster the different types of appliances based on their power consumption patterns.
The idea of analyzing data and creating predictive models have been out there for quite some time. However, only recently was computational power and data available to make AI work well.
The very important aspect of AI and ML is the need to gather the right data and the right quantity. The data in the organization exists in various formats and the first challenge is to get the data in the right format and right quantity. The data is ultimately used to create the right models.
Monica Rogati’s Data Science Hierarchy of Needs pyramid below shows what’s necessary to add intelligence.
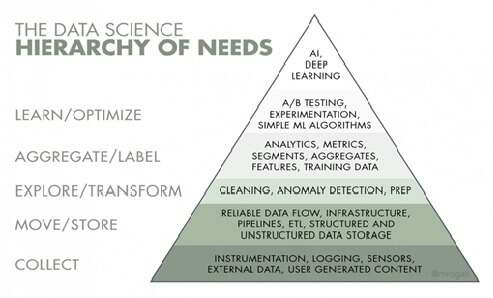
The step in the model regarding data collection is about having the right data sets. The understanding of the data flow is crucial to the successful collection of data sets. After careful cleaning of data and data anomalies, the data is ready for analytics. These were the steps for business intelligence too. What you are building now is predictive modeling or intelligence information based on the data sets. It’s important to have use cases at this stage on what you want to predict or learn from the data in order to build the machine learning models.
AI companies tend to organize data better. Having data organized and centralized in the data warehouse makes it more efficient for engineers or software to exploit that data. Therefore, data gathering and ensuring the data is reliable is critical.
RELATED POST: Artificial Intelligence Governance Part I